Coming Trends to Look Out for in Data Science and Analytics in 2023
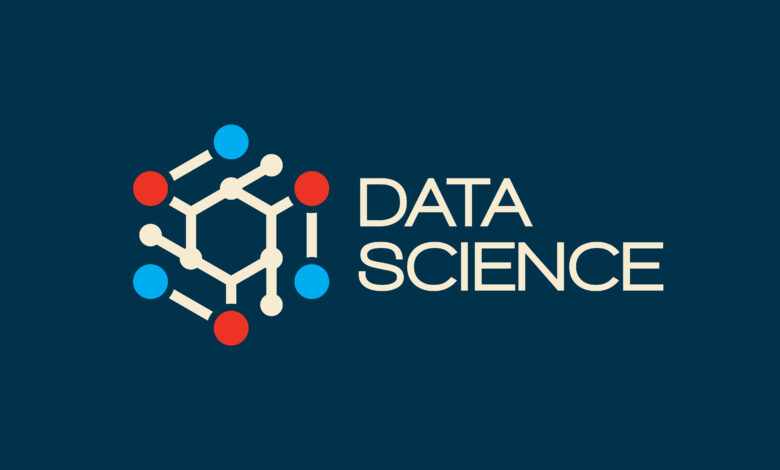
The field of data science and analytics is continually evolving, driven by advancements in technology, increasing data volumes, and the need for businesses to extract actionable insights. As we enter 2023, several exciting trends are set to shape the landscape of data and analytics.
Data analytics lies at the core of nearly every recent innovation. Collecting and analyzing information is often at the heart of each new field – from health care, decentralized work, online shopping, customer support services, or Internet banking to name but a few.
Survey results project that data science and analytics would experience compound annual compound growth of 13.5% between 2020-2030, increasing from an expected value of US$198.08 billion in 2020 to US$684.12 billion by 2030.
From the convergence of artificial intelligence and data analytics to the rise of edge computing and the ethical use of data, organizations must stay abreast of these developments to remain competitive. In this article, we will explore the key trends that are expected to dominate the data and analytics space in 2023.
Data Analytics and Data Science- A Brief
Data science and analytics are two closely related fields that focus on extracting insights and making informed decisions from data. While they share similarities, they also have distinct characteristics. Here’s an overview of data science and analytics:
Data Science:
Data science is an interdisciplinary field that combines elements of statistics, mathematics, programming, and domain knowledge to extract knowledge and insights from data. It involves using scientific methods, algorithms, and procedures to analyze and interpret large and complex datasets. Data science’s main aim is to uncover patterns, trends, and correlations that enable informed decision-making and facilitate solving complex problems.
Data scientists utilize several techniques, including data cleansing and preprocessing, exploratory data analysis, statistical modeling, machine learning, and data visualization. Data scientists utilize several techniques, including data cleansing and preprocessing, exploratory data analysis, statistical modeling, machine learning, and data visualization. They work with structured and unstructured data, often using programming languages like Python or R and utilizing tools and frameworks such as TensorFlow, sci-kit-learn, and PyTorch. Data scientists are also skilled in data storytelling and communicating their findings effectively to stakeholders.
Data Analytics:
Data analytics focuses on using data to gain insights and drive business decisions. It involves the process of collecting, organizing, analyzing, and interpreting data to understand patterns, trends, and relationships. Data analytics often deals with structured data, which is typically organized in databases or spreadsheets.
There are different types of data analytics services, including descriptive analytics (summarizing and visualizing data), diagnostic analytics (examining past data to understand why certain events occurred), predictive analytics (forecasting future outcomes based on historical data), and prescriptive analytics (providing recommendations and strategies to optimize decision-making).
Data analysts employ various statistical and analytical techniques, including data mining, data visualization, regression analysis, and statistical modeling. They use tools like Excel, SQL, Tableau, or Power BI to manipulate and analyze data, generate reports, and communicate insights to stakeholders. The data analytics consultancies offered by the range of data analytics companies are creating a bubble where the need is high and so is the value for the same keeping in mind the future aspects.
Relationship between Data Science and Analytics:
Data science and analytics are closely intertwined and share many common techniques and methodologies. Both fields rely on data to derive insights, employ statistical analysis and modeling, and utilize programming and visualization tools. However, data science often focuses on more advanced techniques, such as machine learning and deep learning, to extract knowledge from complex and unstructured datasets. Data analytics, on the other hand, tends to focus on analyzing historical data to support decision-making and optimize business processes.
In practice, the terms “data science” and “data analytics” are sometimes used interchangeably, and the exact definitions and boundaries between the two can vary depending on the organization and context. Both fields play vital roles in leveraging data to gain actionable insights and drive innovation and efficiency across various industries, making them essential components of the modern data-driven landscape.
Importance of Data Analytics and Data Science
Data science and analytics play a vital role in today’s information-based world. Here are some key reasons highlighting the importance of data science and analytics:
1. Data-Informed Decision-Making:
Data science and analytics provide organizations with the tools and techniques to extract meaningful insights from large volumes of data. By analyzing historical data and recognizing patterns, trends, and correlations within it, businesses can make informed decisions based on evidence rather than intuition or guesswork alone.
Data-driven decision-making leads to improved accuracy, efficiency, and effectiveness across various aspects of an organization’s operations.
2. Competitive Advantage
Today’s business landscape is fiercely competitive; finding ways to stand out is imperative if businesses want to remain viable and succeed. Gaining a competitive advantage is essential if businesses hope to remain relevant. Data science and analytics help organizations recognize market trends, customer preferences, and emerging opportunities to make strategic business decisions.
By leveraging data insights, companies can make strategic decisions that optimize their operations, enhance customer experiences, develop targeted marketing campaigns, and create innovative products and services. This gives them an advantage over competitors who may not be utilizing data-driven approaches.
3. Improved Operational Efficiency
Data science and analytics can uncover inefficiencies, bottlenecks, and areas for optimization within business processes. By analyzing operational data, organizations can identify areas for improvement, streamline workflows, and lower costs.
For example, predictive analytics can be used to forecast demand and optimize supply chain management, resulting in better inventory management and reduced waste. Data-driven insights can also improve resource allocation, enhance productivity, and enable more efficient decision-making at all levels of the organization.
4. Amplified Customer Understanding
Data science and analytics enable organizations to gain a deeper understanding of their customers. By analyzing customer data, such as demographics, preferences, behavior patterns, and feedback, businesses can personalize their products, services, and marketing efforts.
This personalized approach enhances customer satisfaction, loyalty, and retention. Furthermore, data-driven insights can help identify potential customer segments, target new markets, and tailor marketing campaigns to specific audiences, resulting in improved customer acquisition and revenue growth.
5. Risk Management and Fraud Detection
Data science and analytics are instrumental in identifying and managing risks within organizations. By analyzing historical and real-time data, businesses can detect anomalies, patterns, and trends that indicate potential risks or fraudulent activities.
Predictive analytics and machine learning algorithms can flag suspicious transactions, prevent fraud, and protect businesses from financial losses. Additionally, data-driven risk management helps organizations proactively address and mitigate potential risks, ensuring business continuity and safeguarding their reputation.
6. Innovation and Research
Data science and analytics drive innovation and enable scientific research in various domains. By analyzing large and complex datasets, researchers can uncover new insights, validate hypotheses, and make breakthrough discoveries.
Data-driven research helps in fields such as healthcare, genetics, climate science, social sciences, and many others. Data science techniques, such as machine learning and deep learning, contribute to the development of innovative technologies, including artificial intelligence, autonomous systems, and advanced analytics solutions.
In summary, data science and analytics have become critical for organizations across industries. They empower businesses to make data-informed decisions, gain a competitive advantage, improve operational efficiency, understand customers better, manage risks, drive innovation, and contribute to scientific advancements. Embracing data science services is no longer optional but essential for organizations aiming to thrive and succeed in the data-driven era.
Exploring the Key Trends
I. Convergence of Artificial Intelligence and Analytics
Artificial intelligence (AI) and analytics are coming together to drive transformative changes in data-driven decision-making. In 2023, we can expect to see increased integration of AI algorithms and techniques into analytics platforms. This convergence will enable more advanced predictive and prescriptive analytics, as well as enhanced natural language processing and machine learning capabilities.
Organizations will leverage AI-powered analytics to gain deeper insights from complex and unstructured data sources, unlocking hidden patterns and trends that were previously difficult to uncover. The combination of AI and analytics will also lead to the automation of data preparation, analysis, and reporting tasks, saving time and improving accuracy.
II. Edge Computing for Real-Time Analytics
As the volume and velocity of data continue to grow exponentially, there is a growing need for real-time analytics. Edge computing, which brings computation and data storage closer to the data source, is set to play a crucial role in meeting this demand. In 2023, we can expect to see increased adoption of edge analytics, enabling organizations to process and analyze data at the edge of the network.
This approach reduces latency and enables faster decision-making, particularly in time-sensitive applications such as Internet of Things (IoT) devices, autonomous vehicles, and smart manufacturing. Edge computing also addresses concerns around data privacy and security by keeping sensitive data localized and reducing the need for data transfers to centralized servers.
III. Data Governance and Ethical Use of Data
With the growing importance of data in today’s digital economy, data governance, and ethical considerations are gaining prominence. In 2023, organizations will focus on strengthening their data governance frameworks to ensure data quality, compliance with regulations (such as GDPR and CCPA), and ethical use of data. This includes establishing clear data policies, implementing robust data protection measures, and promoting transparency in data collection and usage.
Privacy-enhancing technologies like differential privacy and secure multi-party computation will gain traction, allowing organizations to derive insights from sensitive data without compromising individual privacy. Furthermore, ethical considerations will drive the development of responsible AI practices, including fairness, transparency, and accountability in algorithmic decision-making.
IV. Democratization of Analytics
As data and analytics become increasingly critical for business success, there is a growing emphasis on democratizing analytics capabilities. In 2023, we can expect to see the rise of self-service analytics tools and platforms that empower non-technical users to access and analyze data independently.
These tools will offer intuitive interfaces, drag-and-drop functionality, and natural language querying, enabling business users to derive insights without heavy reliance on data analysts or data scientists. This democratization of analytics will foster a data-driven culture throughout organizations, enabling faster decision-making, improved collaboration, and innovation across departments.
V. Enhanced Data Visualization and Storytelling
Data visualization plays a crucial role in conveying insights effectively. In 2023, we will witness advancements in data visualization techniques, enabling more immersive and interactive experiences. Augmented reality (AR) and virtual reality (VR) technologies will be leveraged to create immersive data visualizations, allowing users to explore data in three-dimensional
environments. Furthermore, storytelling techniques will be integrated into data visualization tools, enabling analysts to create compelling narratives that effectively communicate complex insights to stakeholders. These enhancements in data visualization and storytelling will drive better understanding, engagement, and decision-making based on data.
To Wind Up
As we step into 2023, the world of data and analytics is poised for significant advancements. The convergence of artificial intelligence and analytics, the adoption of edge computing, the focus on data governance and ethical considerations, the democratization of analytics, and the evolution of data visualization techniques are some of the key trends to watch out for.
Organizations that embrace these trends and adapt their strategies and technologies accordingly will gain a competitive edge by harnessing the power of data to drive informed decision-making and unlock new opportunities. Businesses must stay abreast of these trends and leverage them to unlock the full potential of their data assets in the dynamic landscape of data and analytics.