The Role of Differential Equations in Financial Mathematics
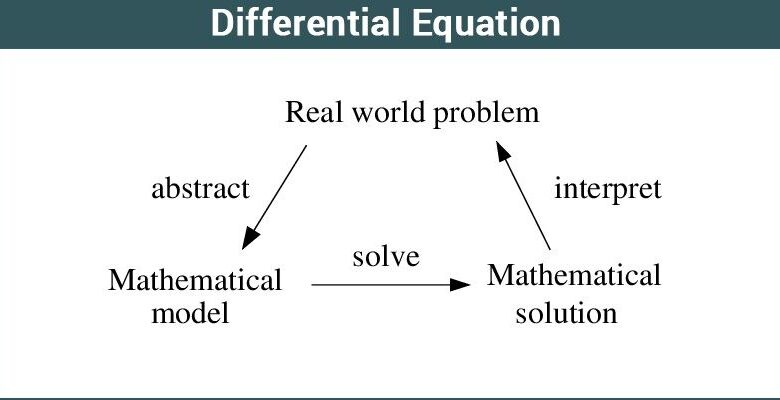
In the realm of financial mathematics, differential equations play a pivotal role in modeling and solving problems related to various financial instruments and markets. These mathematical tools are essential for quantitative finance, enabling analysts and researchers to understand and predict the behavior of financial systems. This article explores the importance of differential equations in financial mathematics, highlighting their applications, types, and significance in the field.
Understanding Differential Equations
Differential equations are mathematical equations that describe the relationship between a function and its derivatives. They are used to model the rates of change in various systems, making them invaluable for studying dynamic processes. In financial mathematics, differential equations help model the evolution of asset prices, interest rates, and other financial variables over time.
There are two main types of differential equations used in financial mathematics: ordinary differential equations (ODEs) and partial differential equations (PDEs). ODEs involve functions of a single variable and their derivatives, while PDEs involve functions of multiple variables and their partial derivatives. Both types are crucial for different aspects of financial modeling.
Applications in Financial Mathematics
- Option Pricing Models
One of the most well-known applications of differential equations in financial mathematics is in option pricing. The Black-Scholes model, a cornerstone of modern quantitative finance, uses a partial differential equation to estimate the price of European call and put options. The Black-Scholes PDE is derived from the stochastic differential equation that describes the dynamics of the underlying asset price. Solving this PDE provides the theoretical price of the option, which is essential for traders and investors. - Interest Rate Models
Interest rate modeling is another area where differential equations are extensively used. Models like the Vasicek model and the Cox-Ingersoll-Ross (CIR) model employ stochastic differential equations to describe the evolution of interest rates over time. These models help in pricing interest rate derivatives, managing interest rate risk, and constructing yield curves. - Portfolio Optimization
Differential equations also play a role in portfolio optimization. The Hamilton-Jacobi-Bellman (HJB) equation, a type of PDE, is used to solve dynamic optimization problems in portfolio management. The HJB equation helps determine the optimal investment strategy by maximizing the expected utility of the portfolio over a given time horizon. - Credit Risk Modeling
In credit risk modeling, differential equations are used to assess the probability of default and the value of credit derivatives. The Merton model, for example, uses a stochastic differential equation to model the value of a firm’s assets and determine the likelihood of default. This model is fundamental for pricing credit default swaps and managing credit risk.
The Significance of Differential Equations in Quantitative Finance
The use of differential equations in quantitative finance is indispensable for several reasons:
- Precision in Modeling
Differential equations provide a precise mathematical framework for modeling the continuous-time dynamics of financial variables. This precision is crucial for accurately estimating prices, risks, and optimal strategies in financial markets. - Flexibility and Adaptability
Differential equations are highly adaptable and can be tailored to model various financial phenomena. Whether it’s the price of an option, the movement of interest rates, or the risk of default, differential equations offer the flexibility to capture the complexities of financial markets. - Foundation for Advanced Techniques
Many advanced techniques in quantitative finance, such as Monte Carlo simulations and numerical methods, rely on differential equations. These techniques are used to approximate solutions to complex financial problems that cannot be solved analytically. Understanding differential equations is therefore essential for implementing and interpreting these advanced methods. - Insight into Financial Dynamics
Differential equations provide insights into the underlying dynamics of financial markets. By studying the solutions to these equations, researchers can gain a deeper understanding of how various factors influence asset prices, interest rates, and other financial variables. This knowledge is invaluable for making informed investment decisions and developing robust financial models.
Challenges and Future Directions
Despite their importance, the use of differential equations in financial mathematics is not without challenges. One of the main challenges is the complexity of solving these equations, especially for high-dimensional problems and non-linear PDEs. Advances in computational methods and numerical techniques are continually being developed to address these challenges and improve the accuracy and efficiency of solutions.
Another challenge is the calibration of models to real-world data. Differential equation models often require parameter estimation, which can be difficult and sensitive to market conditions. Ongoing research in model calibration and parameter estimation is crucial for enhancing the reliability of these models.
Looking ahead, the integration of machine learning with differential equations presents exciting opportunities for financial mathematics. Machine learning algorithms can be used to approximate solutions to differential equations and uncover patterns in financial data that traditional methods might miss. This synergy between machine learning and differential equations has the potential to revolutionize quantitative finance and further enhance our understanding of financial markets.
Conclusion
Differential equations are a cornerstone of financial mathematics, underpinning many models and techniques used in quantitative finance. Their ability to accurately model the dynamics of financial variables makes them indispensable for option pricing, interest rate modeling, portfolio optimization, and credit risk assessment. Despite the challenges, ongoing advancements in computational methods and the integration of machine learning hold promise for the future of differential equations in financial mathematics. As the field continues to evolve, differential equations will remain a fundamental tool for understanding and navigating the complexities of financial markets.