The Rise of Autonomous Medical Coding: Revolutionizing Healthcare Documentation
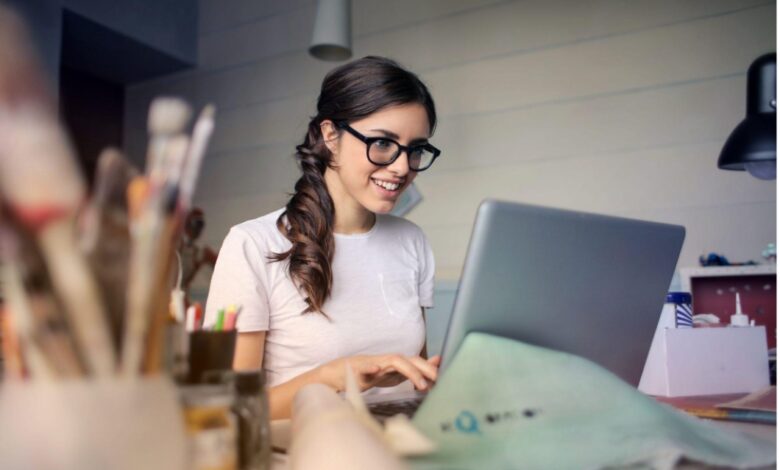
In today’s fast-paced healthcare environment, administrative efficiency is just as critical as clinical excellence. One of the most time-consuming yet essential administrative tasks in healthcare is medical coding—the process of translating clinical diagnoses, procedures, and services into standardized codes used for billing, documentation, and statistical purposes. Traditionally, medical coding has been a manual and error-prone process. However, with the advent of artificial intelligence (AI) and machine learning (ML), a transformative shift is underway: autonomous medical coding.
What is Autonomous Medical Coding?
Autonomous medical coding refers to the use of advanced algorithms and AI systems to automatically assign standardized medical codes to clinical documentation without or with minimal human intervention. Unlike traditional computer-assisted coding (CAC) tools that support human coders, autonomous coding systems can analyze clinical texts, understand context, and independently generate accurate coding outputs.
These systems leverage natural language processing (NLP), deep learning models, and large datasets to “read” electronic health records (EHRs), interpret clinical language, and assign codes from standardized systems such as ICD-10, CPT, and HCPCS.
The Need for Autonomous Coding
The demand for autonomous coding stems from several healthcare industry challenges:
- Shortage of Skilled Coders: The healthcare industry faces a chronic shortage of certified medical coders. Autonomous systems help fill this gap.
- Administrative Burden: Clinicians spend a significant amount of time on documentation and coding. Automating this process allows them to focus more on patient care.
- Error Reduction: Manual coding is prone to inconsistencies and human error. AI systems offer enhanced accuracy and consistency.
- Scalability: As patient volumes grow, especially with aging populations and telehealth expansion, autonomous coding provides a scalable solution for managing documentation efficiently.
How It Works
Autonomous medical coding systems integrate with EHR platforms and use several key technologies:
- Natural Language Processing (NLP): This allows the system to interpret unstructured clinical notes, identifying relevant terms, diagnoses, and procedures.
- Machine Learning (ML): These models learn from large datasets of coded medical records, improving their accuracy over time through supervised and unsupervised learning.
- Contextual Understanding: Advanced AI models can understand clinical context, distinguishing between similar-sounding conditions or procedures based on patient history and physician notes.
- Code Generation and Validation: Once the system determines the appropriate codes, it cross-validates them against payer rules and compliance standards to ensure correctness.
Benefits of Autonomous Medical Coding
The implementation of autonomous coding solutions offers numerous advantages for healthcare providers, payers, and patients:
1. Efficiency and Speed
AI systems can process vast amounts of data rapidly, significantly reducing the turnaround time for coding. This accelerates the revenue cycle, resulting in faster reimbursements.
2. Accuracy and Consistency
With the ability to learn from past data and apply coding guidelines systematically, autonomous coding reduces variability and improves compliance with regulatory standards.
3. Cost Savings
By minimizing reliance on human coders and reducing coding-related denials, organizations can lower administrative costs.
4. Real-time Feedback
Autonomous systems can provide real-time coding suggestions to physicians at the point of care, improving documentation quality and reducing the need for rework.
5. Enhanced Compliance
These systems are programmed to stay updated with the latest coding guidelines, payer rules, and federal regulations, helping healthcare organizations avoid penalties.
Challenges and Considerations
Despite its benefits, autonomous medical coding is not without challenges:
1. Data Privacy and Security
AI systems require access to sensitive patient data, raising concerns about HIPAA compliance and data protection.
2. Integration Complexity
Integrating autonomous coding tools with existing EHR systems and workflows can be technically demanding and resource-intensive.
3. Contextual Limitations
While AI has made strides in understanding language, nuanced clinical decision-making, and ambiguous cases may still require human oversight.
4. Regulatory Oversight
Healthcare is a highly regulated industry. Autonomous systems must be transparent, auditable, and aligned with clinical and legal standards.
5. Resistance to Change
Staff may be hesitant to adopt new technologies, fearing job loss or mistrusting automated decisions. Change management is critical to successful implementation.
Human-AI Collaboration: A Hybrid Model
Most industry experts agree that the near-term future lies in augmented intelligence—a model where AI supports human coders rather than replacing them entirely. In this hybrid approach, AI handles straightforward cases autonomously, while complex or ambiguous cases are flagged for human review.
This ensures both speed and accuracy while maintaining clinical oversight. Coders evolve into quality assurance professionals, focusing on exceptions, training AI models, and auditing for compliance.
Real-World Adoption
Several healthcare organizations have already begun piloting and deploying autonomous coding systems. For example:
- Large hospital systems are using AI to code inpatient encounters, significantly reducing discharge-to-bill times.
- Insurance companies are leveraging these tools to audit claims more effectively and detect fraud.
- Outpatient clinics use real-time coding assistants to improve documentation accuracy during patient visits.
Vendors in this space include established EHR providers integrating AI capabilities, as well as specialized startups focusing solely on autonomous coding platforms.
The Future Outlook
As AI models continue to improve, the scope and accuracy of autonomous coding will expand. Future developments may include:
- Multilingual support for coding in different languages.
- Predictive coding that anticipates diagnoses and procedures based on trends in the patient’s data.
- Voice-to-code technology that allows physicians to dictate notes and receive instant coding suggestions.
Moreover, as autonomous systems become more trusted, we may see their adoption extend to real-time claims submission, automated quality reporting, and even population health analytics.
Conclusion
Autonomous medical coding is reshaping how healthcare organizations manage clinical documentation and revenue cycle management. By leveraging AI, NLP, and machine learning, these systems offer a powerful solution to the longstanding challenges of accuracy, efficiency, and compliance in medical coding.
However, successful adoption requires more than just technology—it demands thoughtful integration, ongoing training, and a shift in how human expertise is applied. As healthcare continues its digital transformation, autonomous medical coding stands out as a critical innovation, enabling clinicians to do what they do best: care for patients